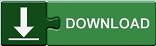

"Power." Wolfram Language & System Documentation Center. Wolfram Research (1988), Power, Wolfram Language function, (updated 2021). Ĭite this as: Wolfram Research (1988), Power, Wolfram Language function, (updated 2021). The special case CubeRoot corresponds to Surd. Exponent expr, form, h applies h to the set of exponents with which form appears in expr. To obtain a real-valued n root, Surd can be used. Exponent expr, form gives the maximum power with which form appears in the expanded form of expr. Because of this branch cut, Power returns a complex root by default instead of the real one for negative real x and odd positive n.

The inverse of a power function is given by Log, so solving the equation for gives a principal solution of. A number to the first power is equal to itself ( ), and 1 to any complex power is equal to 1 ( ). The expression Power is commonly represented using the shorthand syntax x^ y or written in 2D typeset form as x y. Power is a mathematical function that raises an expression to a given power.And with only 18 data points a more complex model with maybe a better fit to the observed data is not justifiable. What does this mean It means the slope is the same as the function value (the y-value) for all points on the graph. The Lyapunov characteristic exponents play a crucial role in the description of the. In doing so one would see that requesting more decimal places in the predictions does not provide a better fit and that only 3 or maybe 4 digits to the right of the decimal are warranted given the quality of the fit. 78 THE MATHEMATICA JOURNAL 1996 Miller Freeman Publications. Using LinearModelFit (or NonlinearModelFit) provides a whole lot more information about the fit than FindFit. However, Mathematicareturns something that doesnt look like that formula and its result is trying to take the square root of a negative number. I expect that the result is Rodrigues rotation formula I + sin(theta)S + (1-cos(theta))SS. Having said all of the above for this particular dataset a better approach that more closely matches the residual error structure is the taking of the logs of the dependent variable and performing a linear regression as Michael Seifert did (but I'd use LinearModelFit rather than NonlinearModelFit - however, in this case the results are equivalent). Im trying to take the matrix exponential of a skew symmetric rotation matrix, S.
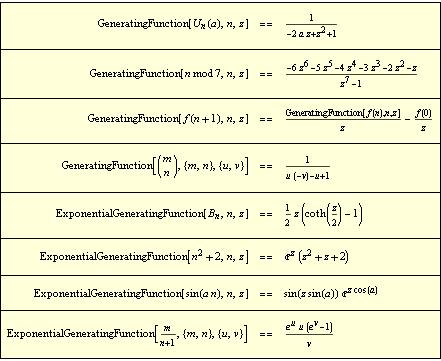
I'm trying to fit the following data using Mathematica, but unfortunately I'm not getting any decent results.
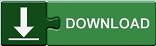